Brianna Posadas and students present research at the AI in Agriculture Conference 2023
February 27, 2023
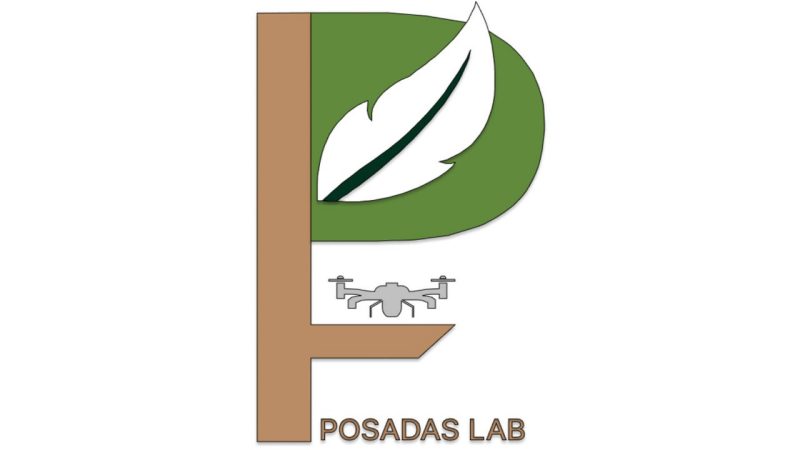
Brianna Posadas (School of Plant and Environmental Science) and her students Sikhar Poudel (School of Plant and Environmental Science), Shreya Mitra (Department of Agricultural, Leadership and Community Education) and Mehul Bhanushali (Department of Computer Science) will present their research at the AI in Agriculture conference 2023. The Posadas Lab, in the School of Plant and Environmental Science, studies the human-technology interface of agricultural technologies: from how to work with agricultural users to make technologies more accessible, to understanding how agricultural users trust and adopt these technologies, and how new technologies can affect agricultural policies.
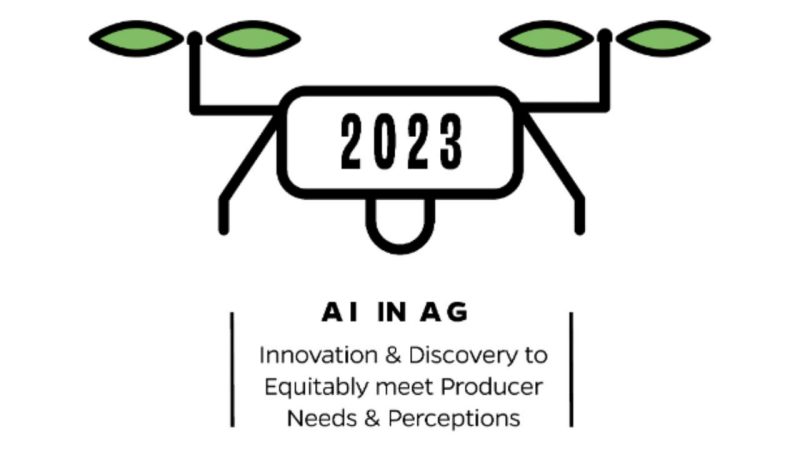
The USDA NIFA AI in Agriculture conference provides a space where university faculty and students, industry, and representatives of different stakeholder groups can share their expertise, vision, and the collective need to develop AI-driven agriculture. The meeting will address multiple aspects of the USDA agriculture innovation agenda and would also be targeting NIFA priority—by building shared-knowledge of our diverse research strengths, creating new networking partnerships, and identifying topic areas where innovation, policy change, or outreach is needed. The 2023 conference is taking place from April 17 to 19 at Orlando, Florida.
Farmers’ User-experience Related to Digital Advancements in Agriculture
Authors: Shreya Mitra, Mehul Bhanushali, Abhilash Chandel, Matthew Chappell, Katheryn Parraga-Estrada, Robin White, Brianna Posadas
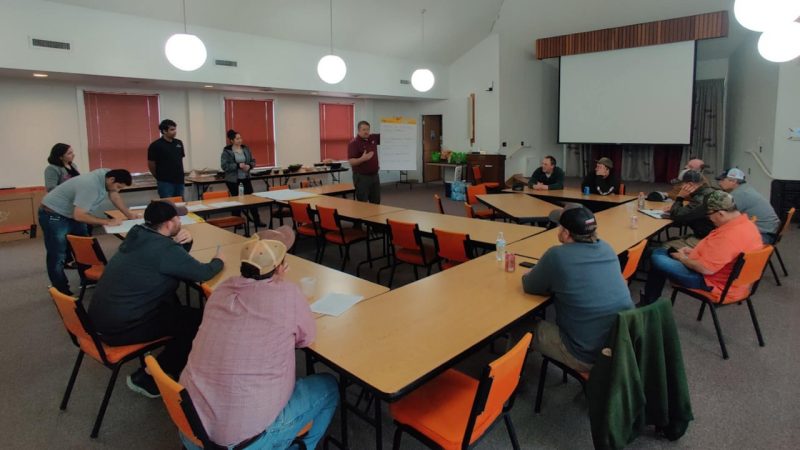
Digital advancements in agriculture are a priority, particularly in developed nations. Literature states that digitalization will be a boon for the agriculture community in general, but not much is known about ground reality, when it comes to farmers' experiences with technology and how it interferes with their agrarian decision-making.
This study highlights farmers' user experience about digital technology usage in agriculture. A focus group was conducted by Virginia Tech at the Tidewater Agricultural Research and Extension Center with farmers as a part of the USDA-funded National Agricultural Producers Data Cooperative (NAPDC) program. Farmers were asked to identify challenges and potential solutions while adopting technologies in agriculture. The challenges pertained to keeping up with evolving technology, determining how to use data for in-farm decision-making, a lack of on-farm connectivity and data protection; lack of support in terms of training and demonstration for technology worthiness; and a lack of cost and infrastructure support.
Plausible solutions highlighted by the respondents included the requirement for third parties to stay up to date on technology, be early adopters and beta testers, as well as provide unbiased and authentic validation reports that highlight economic risks and benefit-cost ratios surrounding technology usage. The respondents also identified the role of universities and government organizations throughout the solution development process, and the need for better commercial network connectivity, or "hotspots," in the field. At the end, focused in-person precision agriculture training programs from extension agents and or universities, and government subsidies for digital advancements were also identified as critical solutions towards adoption of technologies and data-run solutions in farms.
Results from this study will inform technology developers, policymakers, economists, extension workers, university researchers, and government agencies on how to design a strong infrastructure that is inclusive of farmers' requirements and digitally empowers them towards production agriculture.
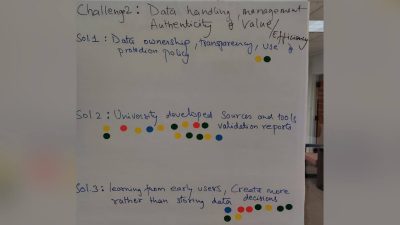
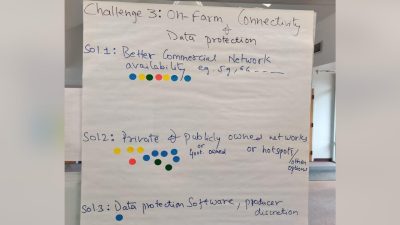
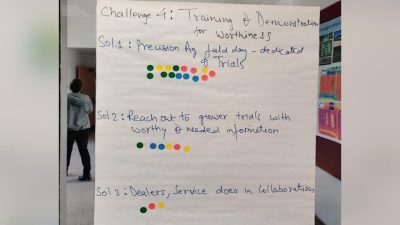
Canopy cover analysis and analysis of the spatial distribution of edamame
Authors: Sikhar Poudel, Song Li, Kshitiz Dhakal, Brianna Posadas
Canopy cover is the vertical projection of the top view of crops in the soil. In previous work, it had been demonstrated that the higher the canopy cover, the higher the yield. Literature states that early canopy cover in the farm gave high yield, and vegetative growth dynamics helped predict soybeans' yield and protein. In addition, the increasing canopy coverage resulted in the suppression of weeds. Furthermore, different genotypes may have variations in the amount of soybean production. A high genetic correlation (0.76) was observed between canopy coverage and soybean grain yield. Canopy cover can be calculated manually in GIS software by making manual grids over aerial images of the crops; this can take up to 3 months. This study examined using automatic grids to calculate canopy cover percentage for edamame.
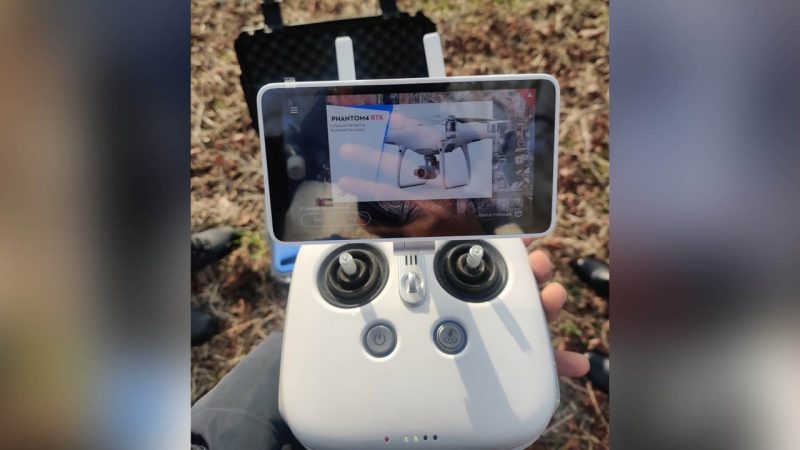
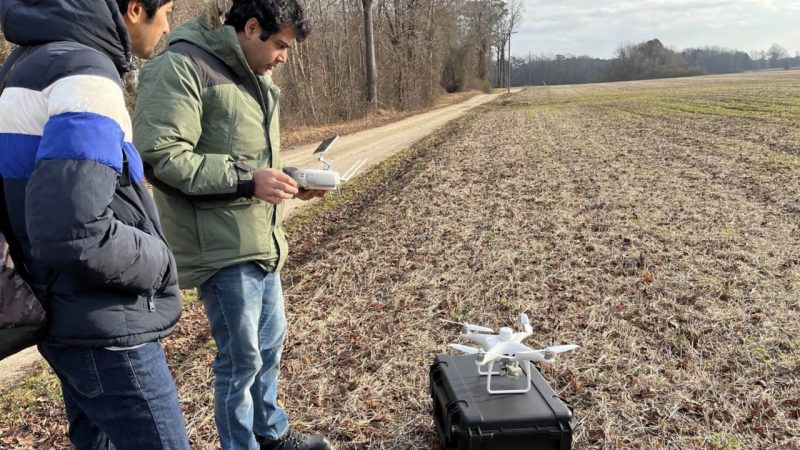
This study expands on previous work where the canopy percentage was calculated for an edamame farm in Virginia that grew 400 different genotypes. On July 6, 2020, drone imagery of edamame was used in this study. The canopy percentage of the farm was first calculated with manual grids using ArcGIS.
In this study, we used QGIS 3.22 Bialowieza long-term release. We segmented the portion of the farm by three criteria: the high canopy area, medium canopy area, and low canopy area. The result from QGIS was used to validate the result from ArcGIS. Here we show that the correlation value was 0.944, the R square value was 0.891, and the P value was less than 0.005. It means the data were statistically significant. This means that the automatic grids in QGIS are a possible quicker alternative method to calculating canopy cover. Additionally, QGIS is an open-source GIS application, which makes this method a more accessible one. Furthermore, the spatial distribution of canopy cover was analyzed in QGIS, and the canopy cover of edamame was less on the sides of the farm and higher in the middle. There may be various reasons for this, such as fewer nutrients, lack of irrigation water, pests, diseases, etc. Therefore, the analysis of spatial distribution in QGIS may be helpful to focus on the specific area of the farm where we see less canopy cover to work on improvement in real time.
With the lower costs and ease of use of drones, capturing drone imagery for precision agriculture has become more accessible. Future work includes user studies with farmers and precision agriculture specialists to improve data interpretation from drone imagery.
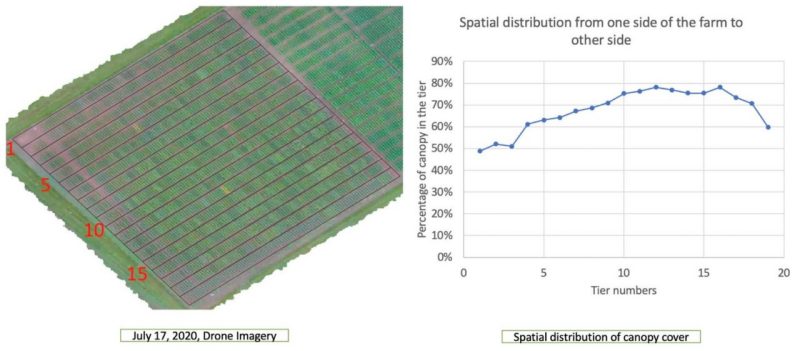
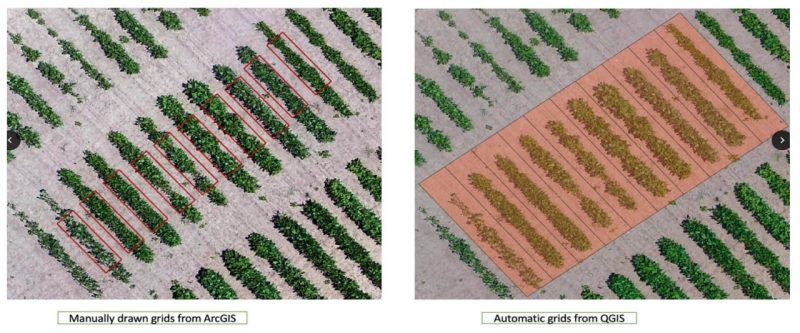