CHCI Participation at IEEE VR 2023
March 20, 2023
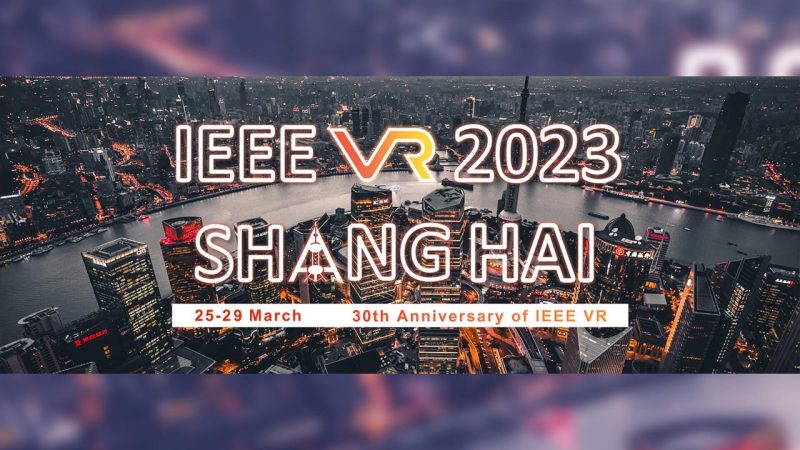
Multiple CHCI faculty and students are participating in the 2023 IEEE Conference on Virtual Reality and 3D User Interfaces (IEEE VR) this month, including Joe Gabbard, Brendan David-John, Jerald Thomas, Doug Bowman, Yalong Yang, Nicholas Polys, Myounghoon Jeon (Philart), John Luksas, Alexander Giovannelli, Shakiba Davari, Ibrahim A. Tahmid, Logan Lane, and Kylie Davidson. IEEE VR is the premier international event for the presentation of research results in the broad areas of virtual, augmented, and mixed reality (VR/AR/MR). IEEE VR 2023, the 30th annual IEEE Conference on Virtual Reality and 3D User Interfaces, will be held from March 25 through 29 in Shanghai, China and online. Virginia Tech, led by CHCI faculty Joe Gabbard, Jerald Thomas, and Doug Bowman, is providing a satellite location in the US for collective remote participation in the conference at the Moss Arts Center in Blacksburg. In addition, CHCI faculty and students have a number of accepted papers, posters, workshops, and contest entries at the conference.
Satellite Event
The satellite event in the Moss Arts Center (MAC), hosted by Doug Bowman, Joe Gabbard, and Jerald Thomas, will bring together approximately 75 researchers from across the US and several international locations to experience the remote conference together, explore collaborations, and build community. In addition to bringing researchers together, the event is a great opportunity to bring visibility to CHCI’s leadership and expertise in the VR/AR/MR space. The satellite event will allow participants to view conference sessions on a 12-hour delay, in order to maintain a standard conference schedule that begins around 8:00 AM local time.
On Monday evening (3/27) conference attendees will be offered local lab tours and demos at multiple sites across campus. Participants will also enjoy a conference dinner on Tuesday evening. Several Virginia Tech graduate students will serve as student volunteers for the satellite event.
Papers
Towards an Understanding of Distributed Asymmetric Collaborative Visualization on Problem-solving
Wai Tong, Meng Xia, Kam Kwai Wong, Doug A. Bowman, Ting-Chuen Pong, Huamin Qu, Yalong Yang
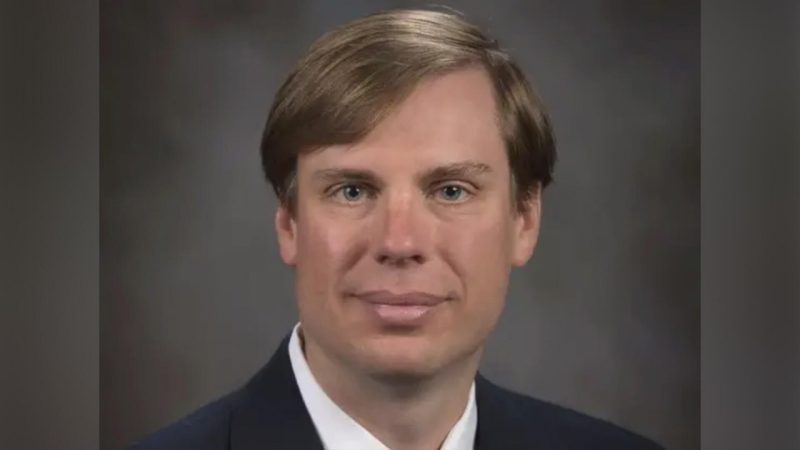
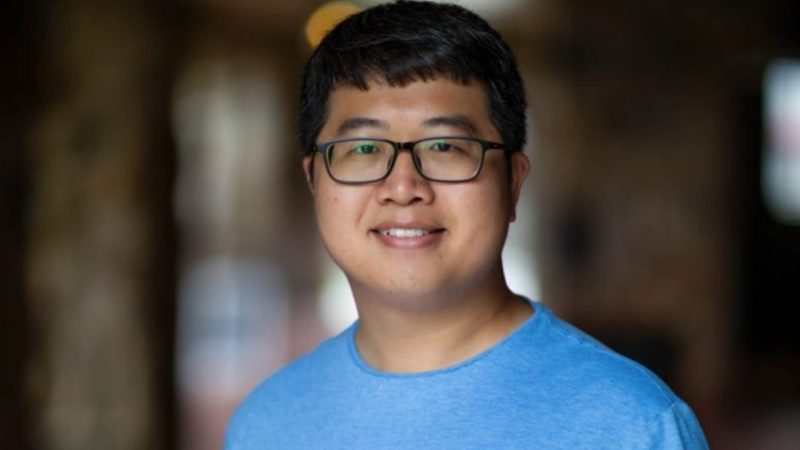
This paper provides empirical knowledge of the user experience for using collaborative visualization in a distributed asymmetrical setting through controlled user studies. With the ability to access various computing devices, such as Virtual Reality (VR) head-mounted displays, scenarios emerge when collaborators have to or prefer to use different computing environments in different places. However, we still lack an understanding of using VR in an asymmetric setting for collaborative visualization.
To get an initial understanding and better inform the designs for asymmetric systems, we first conducted a formative study with 12 pairs of participants. All participants collaborated in asymmetric (PC-VR) and symmetric settings (PC-PC and VR-VR). We then improved our asymmetric design based on the key findings and observations from the first study. Another ten pairs of participants collaborated with enhanced PC-VR and PC-PC conditions in a follow-up study.
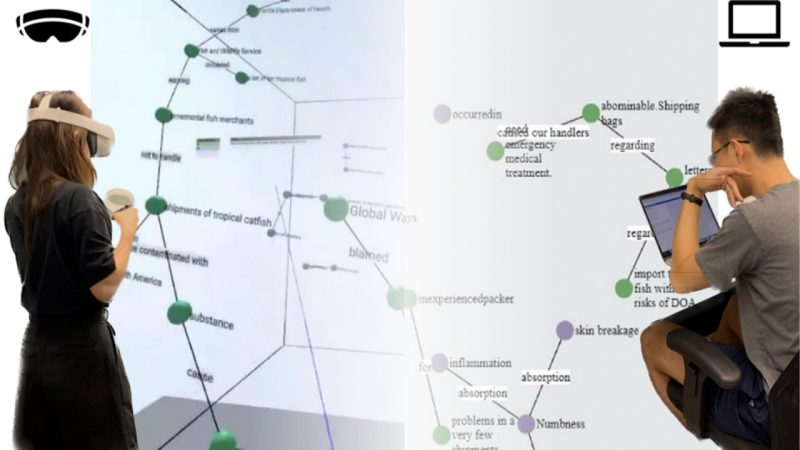
We found that a well-designed asymmetric collaboration system could be as effective as a symmetric system. Surprisingly, participants using PC perceived less mental demand and effort in the asymmetric setting (PC-VR) compared to the symmetric setting (PC-PC). We provide fine-grained discussions about the trade-offs between different collaboration settings.
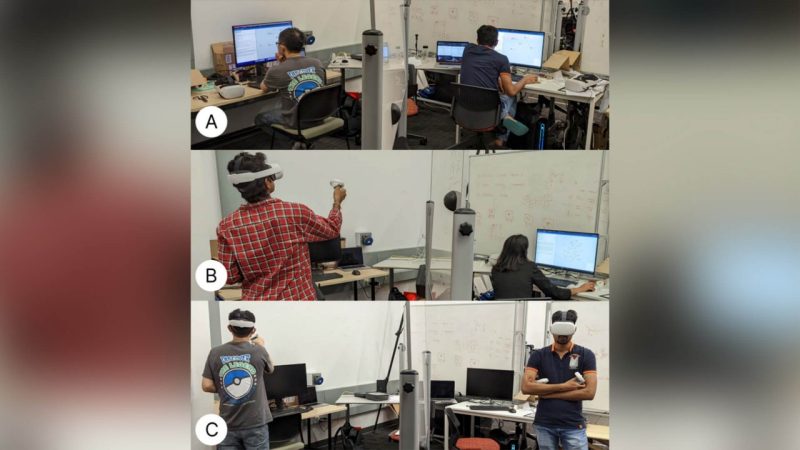
Privacy-preserving Datasets of Eye-tracking Samples with Applications in XR
Brendan David-John, Kevin Butler (University of Florida), Eakta Jain (University of Florida)
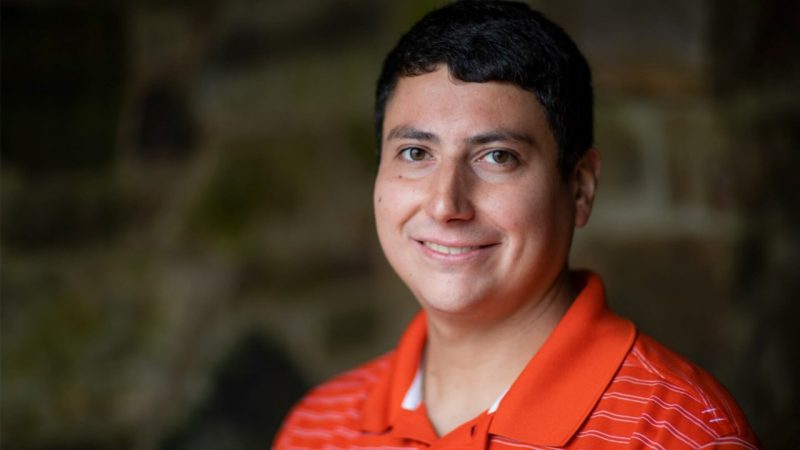
This paper presents methods for processing datasets of eye-tracking data to prevent re-identification of the users that contributed to the dataset. Mechanisms that achieve formal privacy guarantees of k-anonymity and plausible deniability are introduced and compared to the current privacy standard for gaze samples in differential privacy. The presented privacy guarantees provide an upper bound on re-identification risk that protects data even if new identification models are trained by future deep learning approaches.
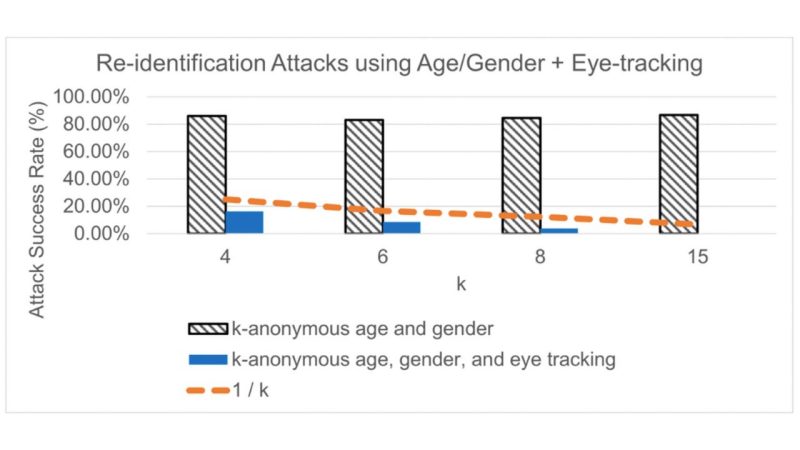
The paper evaluates the loss in utility when privatized datasets are used for machine learning tasks, such as activity recognition and gaze prediction. We find that the privacy-utility trade-offs varied based on task, and recommend plausible deniability or differential privacy when releasing datasets for activity recognition and k-anonymity for gaze prediction datasets.
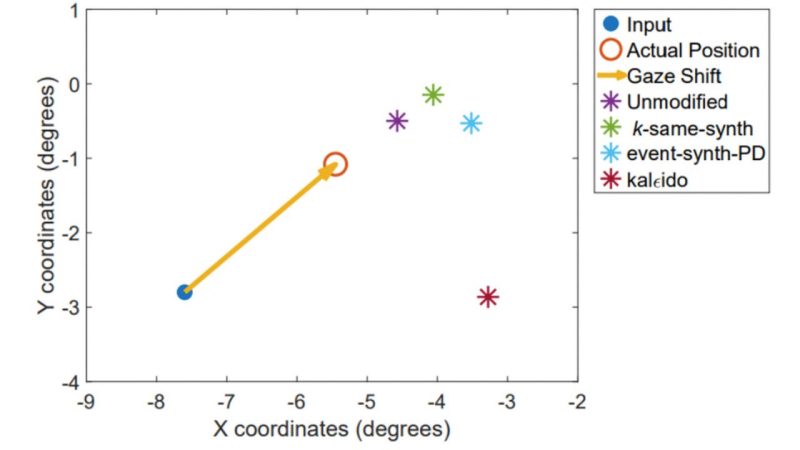
Workshop Papers
Workshop on eXtended Reality for Industrial and Occupational Support (XRIOS)
This workshop aims to identify the current state of XR research and the gaps in the scope of human factors and ergonomics, mainly related to the industrial and occupational tasks, and discuss potential future research directions. The workshop will build a community that bridges XR developers, human factors, and ergonomics researchers interested in industrial and occupational applications, while providing an opportunity for academic and industry researchers to present their latest work or research in progress.
An overview of the 2nd international workshop on extended reality for industrial and occupational supports (XRIOS).
Kim, K., Marques, B., Jeong, H., Silva, S., Cho, I., Ferreira, C., Kim, H., Dias, P., Jeon, M., & Santos, B. S.
Workshop on Immersive Visualization Laboratories - Past, Present and Future
The goal of this workshop is to gather practitioners from immersive visualization laboratories to share their success stories, information about their hardware setups and the software they used and/or developed. Discussion can also include "not-so-successful" stories with lessons learned and workshop participants will also come together to discuss the future of large-scale immersive visualization labs. We also hope to bring visualization practitioners together to advance the way our field works with immersive visualization hardware and software frameworks for a sustainable immersive visualization laboratory.
25 years so far: Lessons from a Large Scale Immersive Visualization Facility
Nicholas Polys and Jayesh Pandey
This article reflects on the challenges and successes of providing cutting-edge immersive visualization facilities for a variety of academic users. For over 25 years, the University Visualization and Animation Group (now Advanced Research Computing) has provided cyberinfrastructure and support for designers, engineers, and scientists with data and visualization needs. We consider the history and evolution of these services and inventory several aspects of their research and educational impact. While there are many other examples of successful installations, we present evidence demonstrating the cross-cutting value of these institutional cyberinfrastructure resources and our prospectives for future research.
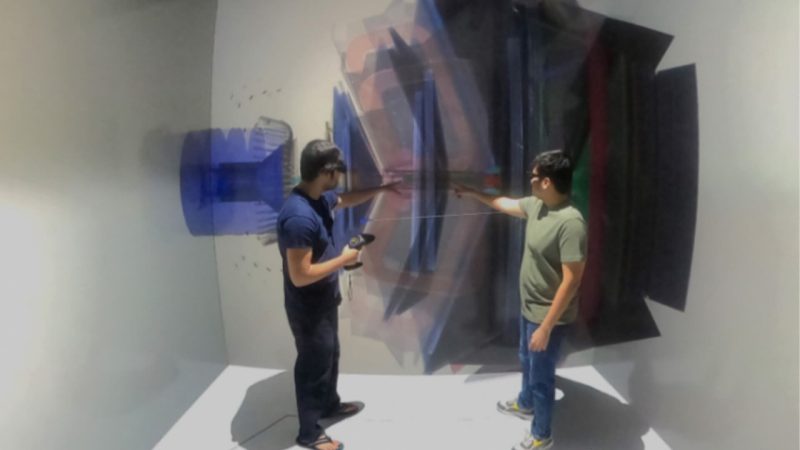
3DUI Contest Entry
CLUE HOG: An Immersive Competitive Lock-Unlock Experience using Hook On Go-Go Technique for Authentication in the Metaverse
Alexander Giovannelli, Francielly Rodrigues, Shakiba Davari, Ibrahim A. Tahmid, Logan Lane, Cherelle Connor, Kylie Davidson, Gabriella N. Ramirez, Brendan David-John, and Doug A. Bowman
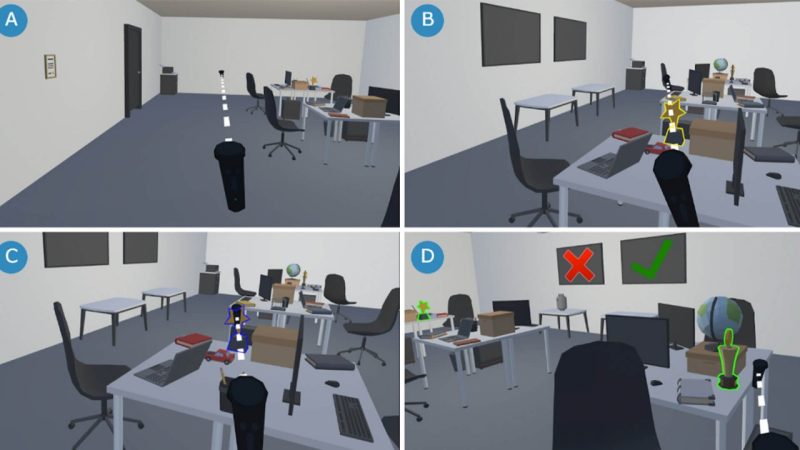
Poster
Interaction-Triggered Estimation of AR Object Placement on Indeterminate Meshes
John Luksas and Joseph L. Gabbard
Current Augmented Reality devices rely heavily on live environment mapping to provide convincing world-relative experiences through user interaction with the real world. This
mapping is obtained and updated through many different algorithms but often contains holes and other mesh artifacts when generated in less ideal scenarios, like outdoors and with fast
movement. In this paper, we present the Interaction-Triggered Estimation of AR Object Placement on Indeterminate Meshes, a work-in-progress application providing a quick, interaction-triggered method to estimate the normal and position of missing mesh in real-time with low computational overhead. We achieve this by extending the user's hand using a group of additional raycast sample points, aggregating results according to different algorithms, and then using the resulting values to place an object.
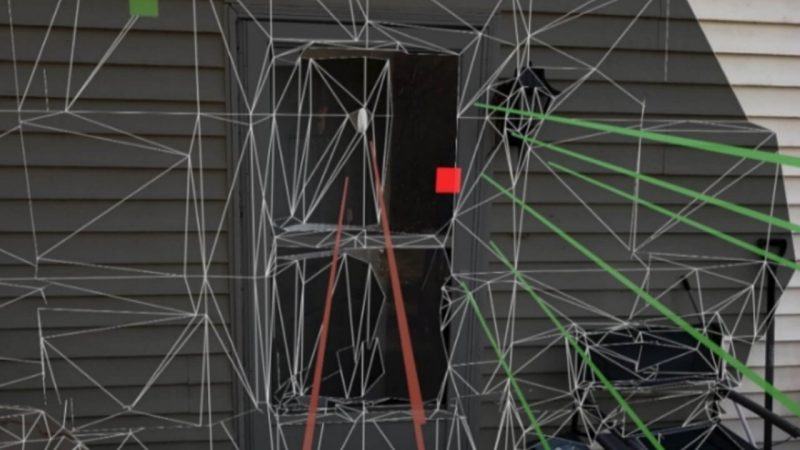