Significant CHCI contributions at CHI 2022
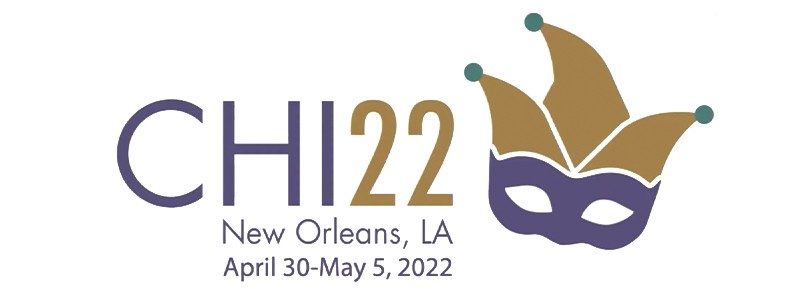
There are significant contributions at the ACM CHI Conference on Human Factors in Computing Systems 2022 from CHCI faculty, students, and alumni. CHI is an international conference of HCI held annually which brings together researchers and practitioners from all over the world and from diverse cultures, backgrounds, and positionalities, who have as an overarching goal to make the world a better place with interactive digital technologies. CHI 2022 is structured as a Hybrid-Onsite full conference from April 30–May 5 in New Orleans, LA. CHCI faculty, students, and alumni won two awards, and will present seven full papers, four workshop papers, and late breaking work, and lead a workshop at the conference.
CHCI members (current or former) are listed in BOLD.
Awards
Honorable Mention Papers
Scott McCrickard (Associate Professor, Computer Science), Lindah Kotut (VT Alumna)
Winds of Change: Seeking, Preserving, and Retelling Indigenous Knowledge Through Self-Organized Online Communities
Md Momen Bhuiyan (Ph.D. Student, Computer Science), Carlos Augusto Bautista Isaza (Ph.D. Student, Computer Science), Tanushree Mitra (former VT CHCI, now University of Washington), Sang Won Lee (Assistant Professor, Computer Science)
OtherTube: Facilitating Content Discovery and Reflection by Exchanging YouTube Recommendations with Strangers
Papers
Yalong Yang (Assistant Professor, Computer Science), Wenyu Xia, Fritz Lekschas, Carolina Nobre, Robert Krueger, and Hanspeter Pfister.
The Pattern is in the Details: An Evaluation of Interaction Techniques for Locating, Searching, and Contextualizing Details in Multivariate Matrix Visualizations
Matrix visualizations are widely used to display large-scale network, tabular, set, or sequential data. They typically only encode a single value per cell, e.g., through color. However, this can greatly limit the visualizations’ utility when exploring multivariate data, where each cell represents a data point with multiple values (referred to as details). Three well-established interaction approaches can be applicable in multivariate matrix visualizations (or MMV): focus+context, pan&zoom, and overview+detail. However, there is little empirical knowledge of how these approaches compare in exploring MMV. We report on two studies comparing them for locating, searching, and contextualizing details in MMV. We first compared four focus+context techniques and found that the fisheye lens overall outperformed the others. We then compared the fisheye lens, to pan&zoom and overview+detail. We found that pan&zoom was faster in locating and searching details, and as good as overview+detail in contextualizing details.
Kun-Ting Chen, Tim Dwyer, Yalong Yang (Assistant Professor, Computer Science), Benjamin Bach, and Kim Marriott.
GAN'SDA Wrap: Geographic And Network Structured DAta on surfaces that Wrap Around
There are many methods for projecting spherical maps onto the plane. Interactive versions of these projections allow the user to center the region of interest. However, the effects of such interaction have not previously been evaluated. In a study with 120 participants we find interaction provides significantly more accurate area, direction and distance estimation in such projections. The surface of 3D sphere and torus topologies provides a continuous surface for uninterrupted network layout. But how best to project spherical network layouts to 2D screens has not been studied, nor have such spherical network projections been compared to torus projections. Using the most successful interactive sphere projections from our first study, we compare spherical, standard and toroidal layouts of networks for cluster and path following tasks with 96 participants, finding benefits for both spherical and toroidal layouts over standard network layouts in terms of accuracy for cluster understanding tasks.
Lindah Kotut (VT Alumna) and Scott McCrickard (Associate Professor, Computer Science)
Winds of Change: Seeking, Preserving, and Retelling Indigenous Knowledge Through Self-Organized Online Communities
Technology has provided an environment for connecting indigenous community members and a means for them to seek and engage with their indigenous knowledge (IK). Emerging research has examined the effects of social media on specific IK, including the possibility of undermining community agency. In this work, we contrast how indigenous community members engage with IK offline, and in their own self-organized communities online. Through interviews with community members and a study of Facebook Pages and Facebook Groups, we seek to better understand these practices and elicit design recommendations. Our findings describe how community roles have shifted in the presence of technology, notably with absence of elders and the inclusion of ``born towns''--community members who live in non-traditional settings. We also find that fluency in the indigenous language serves both as a gatekeeper: guarding community knowledge, while also facilitating discussion surrounding different aspects of IK.
Feiyu Lu (Ph.D. Student, Computer Science) and Yan Xu
Exploring Spatial UI Transition Mechanisms with Head-Worn Augmented Reality
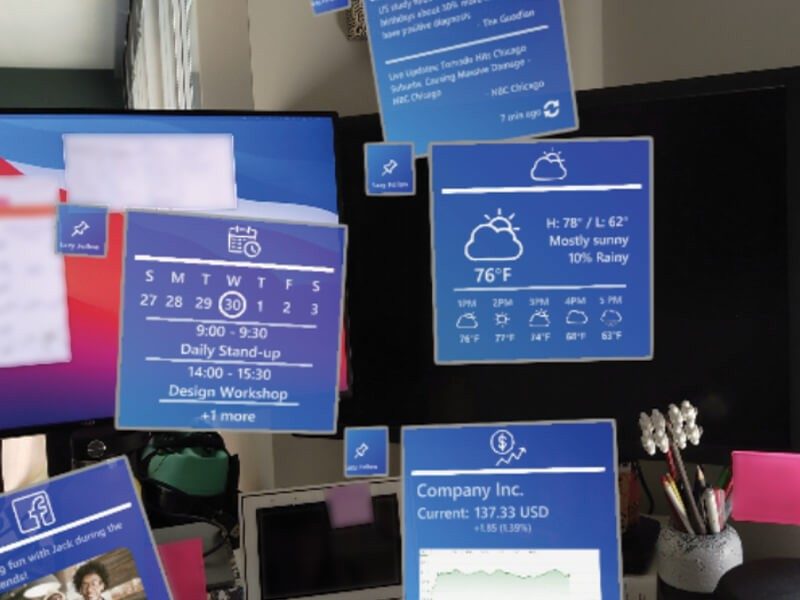
Imagine in the future people comfortably wear augmented reality (AR) displays all day, how do we design interfaces that adapt to the contextual changes as people move around? In current operating systems, the majority of AR content defaults to staying at a fixed location until being manually moved by the users. However, this approach puts the burden of user interface (UI) transition solely on users. In this paper, we first ran a bodystorming design workshop to capture the limitations of existing manual UI transition approaches in spatially diverse tasks. Then we addressed these limitations by designing and evaluating three UI transition mechanisms with different levels of automation and controllability (low-effort manual, semi-automated, fully-automated). Furthermore, we simulated imperfect contextual awareness by introducing prediction errors with different costs to correct them. Our results provide valuable lessons about the trade-offs between UI automation levels, controllability, user agency, and the impact of prediction errors.
Md Momen Bhuiyan (Ph.D. Student, Computer Science), Carlos Augusto Bautista Isaza (Ph.D. Student, Computer Science), Tanushree Mitra (former VT CHCI, now University of Washington), Sang Won Lee (Assistant Professor, Computer Science)
OtherTube: Facilitating Content Discovery and Reflection by Exchanging YouTube Recommendations with Strangers
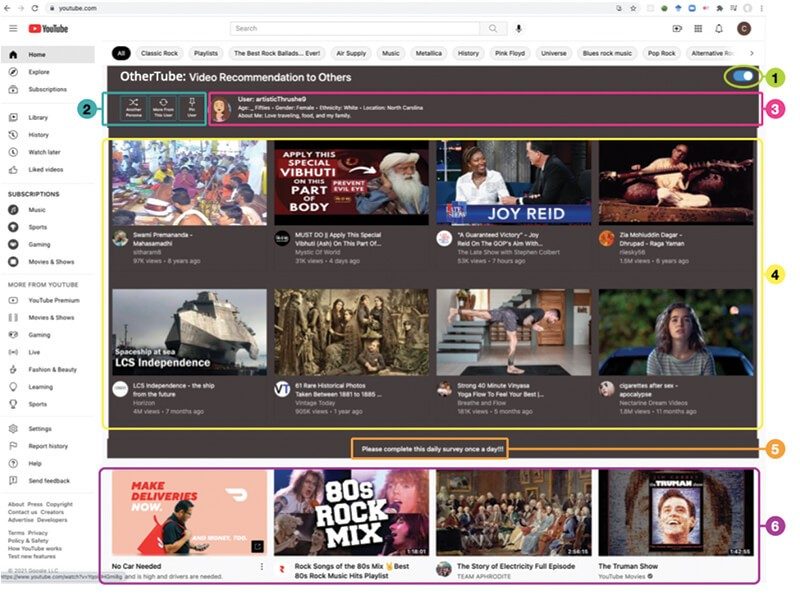
To promote engagement, recommendation algorithms on platforms like YouTube increasingly personalize users' feeds, limiting users' exposure to diverse content and depriving them of opportunities to reflect on their interests compared to others'. In this work, we investigate how exchanging recommendations with strangers can help users discover new content and reflect. We tested this idea by developing OtherTube---a browser extension for YouTube that displays strangers' personalized YouTube recommendations. OtherTube allows users to (i) create an anonymized profile for social comparison (#3 in the figure), (ii) share their recommended videos with others (#6 in the figure), and (iii) browse strangers' YouTube recommendations (#4 in the figure). We conducted a 10-day-long user study (n=41) followed by a post-study interview (n=11). Our results reveal that users discovered and developed new interests from seeing OtherTube recommendations. We identified user and content characteristics that affect interaction and engagement with exchanged recommendations; for example, younger users interacted more with OtherTube, while the perceived irrelevance of some content discouraged users from watching certain videos. Users reflected on their interests as well as others', recognizing similarities and differences. Our work shows promise for designs leveraging the exchange of personalized recommendations with strangers.
Aditya Bharadwaj (VT Alumnus), David Gwizdala (VT Alumnus), Yoonjin Kim (VT Alumnus), Kurt Luther (Associate Professor, Computer Science), T.M. Murali (Professor, Computer Science)
Flud: A Hybrid Crowd–Algorithm Approach for Visualizing Biological Networks
Modern experiments in many disciplines generate large quantities of network (graph) data. Researchers require aesthetic layouts of these networks that clearly convey the domain knowledge and meaning. However, the problem remains challenging due to multiple conflicting aesthetic criteria and complex domain-specific constraints. In this paper, we present a strategy for generating visualizations that can help network biologists understand the protein interactions that underlie processes that take place in the cell. Specifically, we have developed Flud, a crowd-powered system that allows humans with no expertise to design biologically meaningful graph layouts with the help of algorithmically generated suggestions. Further, we propose a novel hybrid approach for graph layout wherein crowd workers and a simulated annealing algorithm build on each other's progress. A study of about 2,000 crowd workers on Amazon Mechanical Turk showed that the hybrid crowd-algorithm approach outperforms the crowd-only approach and state-of-the-art techniques when workers were asked to lay out complex networks that represent signaling pathways. Another study of 7 participants with biological training showed that Flud layouts are more effective compared to those created by state-of-the-art techniques. We also found that the algorithmically generated suggestions guided the workers when they are stuck and helped them improve their score. Finally, we discuss broader implications for mixed-initiative interactions in layout design tasks beyond biology.
Yasmine Belghith (VT Alumna), Sukrit Venkatagiri (Ph.D. Student, Computer Science), and Kurt Luther (Associate Professor, Computer Science)
Compete, Collaborate, Investigate: Exploring the Social Structures of Open Source Intelligence Investigations
Online investigations are increasingly conducted by individuals with diverse skill levels and experiences, with mixed results. Novice investigations often result in vigilantism or doxxing, while expert investigations have greater success rates and fewer mishaps. Many of these experts are involved in a community of practice known as Open Source Intelligence (OSINT), with an ethos and set of techniques for conducting investigations using only publicly available data. Through semi-structured interviews with 14 expert OSINT investigators from nine different organizations, we examine the social dynamics of this community, including the collaboration and competition patterns that underlie their investigations. We also describe investigators’ use of and challenges with existing OSINT tools, and implications for the design of social computing systems to better support crowdsourced investigations.
Late-Breaking Work
Chihab Nadri, Ignacio Alvarez, Esther Bosch, Michael Oehl, Michael Braun, Jennifer Healey, Christophe Jallais, Wendy Ju, Jingyi Li, Myounghoon (Philart) Jeon (Associate Professor, Industrial and Systems Engineering)
Empathic vehicle design: Use cases and design directions from two workshops
Empathic vehicles are expected to improve user experience in automated vehicles and to help increase user acceptance of technology. However, little is known about potential real-world implementations and designs using empathic interfaces in vehicles with higher levels of automation. Given advances in affect detection and emotion mitigation, we conducted two workshops (N1 =24, N2 = 22, Ntotal = 46) on the design of empathic vehicles and their potential utility in a variety of applications. This paper recapitulates key opportunities in the design and application of empathetic interfaces in automated vehicles which emerged from the two workshops hosted at the ACM AutoUI conferences.
Workshops
Barrett Ens, Maxime Cordeil, Chris North (Professor, Computer Science), Tim Dwyer, Lonni Besançon, Arnaud Prouzeau, Jiazhou Liu, Andrew Cunningham, Adam Drogemuller, Kadek Anaa Satriadi, and Bruce H. Thomas
Immersive Analytics 2.0: Spatial and Embodied Sensemaking
Immersive Analytics is now a fully emerged research topic that spans several research communities, including Human-Computer Interaction, Data Visualization, Virtual Reality and Augmented Reality. Immersive Analytics research has identified and validated benefits of using embodied, 3D spatial immersive environments for visualization and have shown benefits in the effective use of space and 3D interaction to explore complex data. As of today, most studies in Immersive Analytics have focused on exploring novel visualization techniques in 3D embodied immersive environments. Thus far, there is a lack of fundamental study in this field that clearly compares immersive versus non immersive platforms for analytics purposes, and firmly delineates the benefits of immersive environments for analytic tasks. We feel that it is time to establish an agenda to assess the benefits and potential of immersive technologies, spatial interfaces, and embodied interaction to support sensemaking, data understanding, and collaborative analytics. This workshop will aim at putting this agenda together, by gathering international experts from Immersive Analytics and related fields to define which studies need to be conducted to assess the effect of embodied interaction on cognition in data analytics.
Workshop Papers
Neelma Bhatti (Ph.D. student, Computer Science), Scott McCrickard and Aisling Kelliher. Connecting in a Pandemic: Using Amazon glow device for interaction over a distance. Paper for the Workshop “Tangible Interaction for Well-being.”
Morva Saaty (Ph.D. student, Computer Science), Jaitun V. Patel (M.S. student, Computer Science), Derek Haqq (Ph.D. student, Computer Science), Tim Stelter (VT Alumni), and Scott McCrickard. Integrating Social Media into the Design Process, Paper for the Workshop “InContext: Futuring User-Experience Design Tools.”
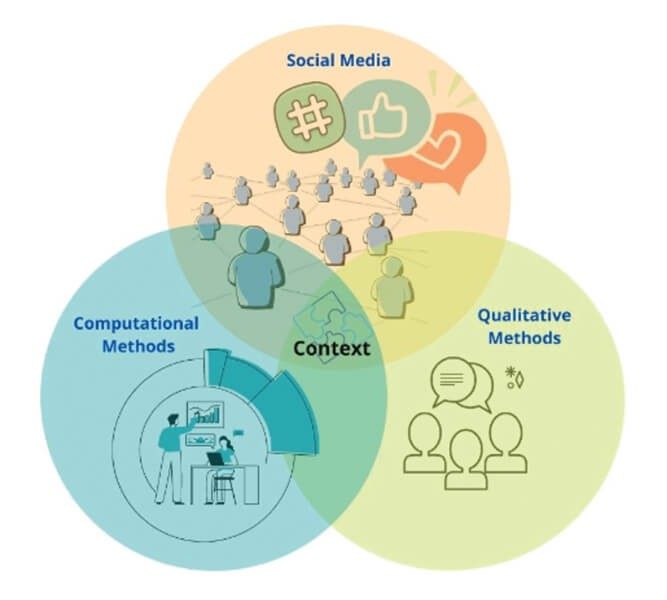
Daniel Manesh (Ph.D. student, Computer Science), Mohammad Khalid, Homero Murzi, Ibukun Awolusi, Abiola Akanmu (Assistant Professor, College of Engineering), Sang Won Lee. Octave: Designing a Data Science Environment for Domain Experts. Paper for the Workshop "Interrogating human-centered data science: Taking stock of opportunities and limitations" https://sites.google.com/view/hcds-workshop-chi2022/home
Anirban Mukhopadhyay (Ph.D. Student, Computer Science), Sukrit Venkatagiri (Ph.D. Student, Computer Science), and Kurt Luther (Associate Professor, Computer Science). Towards designing a flexible expert-led crowdsourcing framework for investigating misinformation. Paper for the Workshop “Designing Credibility tools to combat Mis/Disinformation: A Human-Centered Approach” https://sites.google.com/view/design4misinformation/home